Practical Decision Tree Applications in Business
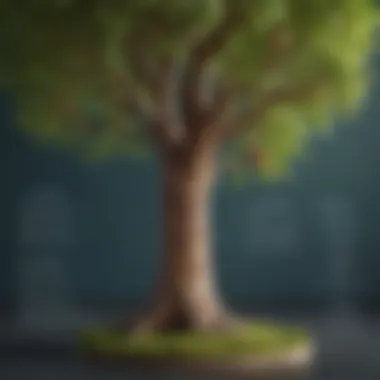
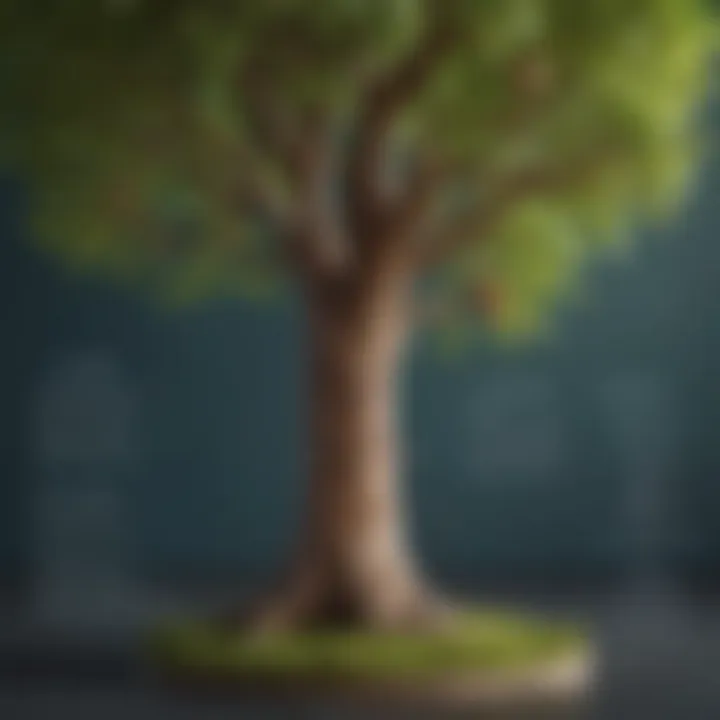
Intro
In the fast-paced world of business, making the right decisions is like walking a tightrope. One misstep can lead to significant consequences, making it essential to employ effective tools in the decision-making process. Decision trees have emerged as a powerful method for navigating the complexities of business choices. These visual representations of possible optionsâalong with their potential outcomesâenable managers and strategists to weigh risks and benefits systematically. This article sets out to explore various examples of how decision trees can be effectively employed across different sectors, enhancing clarity and focus in their strategies.
Investment Dictionaries
Understanding the terms associated with decision trees can empower professionals to implement them more effectively. Whether you are an investor, financial analyst, or a student diving into the business realm, grasping foundational terminology is essential.
Common Financial Terms
- ROI (Return on Investment): A measure used to evaluate the efficiency of an investment, calculated by dividing net profit by total investment cost.
- NPV (Net Present Value): The difference between the present value of cash inflows and outflows over a period of time. This helps in assessing investment profitability.
- Cash Flow: Refers to the net amount of cash and cash-equivalents being transferred in and out of business.
Advanced Investment Terminology
- Sensitivity Analysis: This technique assesses how different values of an independent variable affect a particular dependent variable under a given set of assumptions. It's crucial when utilizing decision trees to understand how various factors influence outcomes.
- Scenario Planning: This involves creating and analyzing different future scenarios to understand potential risks and opportunities better. Decision trees can play a vital role in illustrating these scenarios and guiding decisions.
"In the realm of decision-making, a clear understanding of terms is just as crucial as the decisions themselves."
Expert Advice
Once foundational knowledge is established, navigating the complexities of financial decision-making requires expertise. Rather than relying solely on intuition, integrating data-driven approaches can yield better outcomes.
Tailored Investment Strategies
- Market Analysis: Understand your particular market conditions through a decision tree that maps out potential market changes and the corresponding investment strategies.
- Portfolio Diversification: Utilize decision trees to assess the risk versus return of diversifying your portfolio against market conditions, offering a visual representation of your investment paths.
Risk Management Techniques
- Risk Assessment Models: Use decision trees to visualize various risks associated with each option. This helps prioritize which areas need mitigation and which ones can be strategically embraced.
- Contingency Planning: Build branches in your decision tree for potential setbacks, ensuring you have backup plans ready.
By integrating these concepts and examples into practical scenarios, business professionals can strengthen their approach to critical decision-making. Familiarity with specific terms and strategies enhances the overall efficacy of decision trees, making them invaluable tools in todayâs competitive business landscape.
Prelude to Decision Trees
Decision trees serve as powerful tools in the domain of decision-making, translating intricate scenarios into simpler, more manageable formats. They help businesses distill complex issues into a series of logical choices, facilitating clarity in decision-making processes. As the business landscape grows increasingly driven by data, understanding decision trees becomes essential, especially when navigating through potential risks, uncertainties, and strategic planning.
By using decision trees, organizations can visualize options and ramifications, laying out courses of action that can impact profitability, operational efficiency, and customer satisfaction. In a world where rapid changes keep business leaders on their toes, these trees offer a tree-like model of decisions, outlining branches that represent potential choices and their associated outcomes.
Moreover, decision trees are not merely a theoretical exercise; they contain various practical applications across different industries. From streamlining healthcare diagnostics to enhancing investment strategies in finance, the role of decision trees is wide-ranging and significant. Now, let's explore the foundational aspects of decision trees, starting with the basics.
Understanding the Basics
At its core, a decision tree is a graph that represents choices and their possible consequences laid out in a branching format. Each internal node represents a decision point, while leaf nodes indicate outcomes. This simplicity allows decision trees to be easily interpreted, even by those without a data science background.
A few critical elements characterize decision trees:
- Root Node: This is the starting point of the tree, representing the initial decision or question.
- Branches: The lines connecting nodes serve as pathways leading to new questions or decisions.
- Leaves: These are the endpoints that signify the results or decisions that can be made based on preceding nodes and branches.
Decision trees can be divided into two primary types: classification trees and regression trees. Classification trees are typically used for categorical outcomes, whereas regression trees are suited for continuous outcomes. Though powerful, it's crucial to recognize that the effectiveness of decision trees hinges upon the quality of the data used to construct them and their organizational application.
Historical Context and Development
The concept of decision trees is not as modern as one might think. Their roots trace back to the 1960s, primarily in the fields of statistics and artificial intelligence. The significant development of decision tree algorithms began with the introduction of the ID3 algorithm by Ross Quinlan in 1986. That simple yet effective framework paved the way for subsequent iterations and refinements, such as the C4.5 algorithm and CART (Classification and Regression Trees).
Over the years, organizations began to see the potential of decision trees as they realized their usefulness in risk assessment and predictive modeling. The 1990s saw an exponential rise in their application, particularly in the financial services sector. Decision trees became essential not just for analytics but also for strategic decision-making, helping companies navigate through regulatory environments and economic uncertainties.
With the advent of machine learning and artificial intelligence, the capabilities of decision trees expanded even further. They can now be integrated with ensemble methodsâlike Random Forestsâenhancing their performance and accuracy to tackle more complex datasets.
As we venture through this article, understanding this historical backdrop will enrich the appreciation for how decision trees evolved into indispensable tools for contemporary business strategy and decision-making.
The Structure of Decision Trees
When dissecting decision trees in a business context, understanding their structure is crucial. The layout not only serves as the foundation for effective decision-making but also enhances clarity and creates a visual flow of information. A well-organized decision tree can simplify complex scenarios, allowing stakeholders to grasp outcomes quickly and efficiently, which aids in strategic planning. It helps businesses to visualize choices and their potential impacts, guiding leaders through an efficient decision-making process.
Key Components Explained
At the heart of a decision tree are its key components: nodes, branches, and leaves. Nodes represent decisions or splits based on certain criteria. Each branch emanates from a node, symbolizing the possible choices or outcomes resulting from that decision. Finally, the leaves depict the final choices or outcomes, marking the end of the decision-making path.
These components work together to create a clear roadmap for tackling problems, making complex decisions more digestible. Additionally, weaknesses in the tree structure can lead to misinterpretations, resulting in missed opportunities or misguided strategies. Therefore, a well-thought-out structure is paramount.
Types of Decision Trees
Decision trees can broadly be categorized into two main types: classification trees and regression trees. Each type serves distinct purposes, enabling businesses to apply them according to their specific needs.
Classification Trees
Classification trees are designed to categorize data into discrete classes. They are particularly useful when the outcome variable is categorical, such as determining whether a loan application is approved or denied. A key characteristic of classification trees is their ability to handle qualitative data, making them a favored choice among businesses looking to make categorical decisions.
One unique feature of classification trees is their method of handling uncertainty. They utilize techniques to minimize error, improving the reliability of the decisions made from the tree. However, while they are beneficial due to their straightforward visualization, they can struggle with overfitting when presented with a small dataset. This means that while they might excel in training data, their predictive power can diminish when applied to new data.
Regression Trees
In contrast, regression trees are focused on predicting continuous numerical outcomes. For instance, they could be employed to estimate future sales based on past performance metrics. The primary characteristic of regression trees is their reliance on numerical data, which allows for precise estimations.
One might say a distinct advantage of regression trees is their simplicity in showing relationships between variables. They break down the data into segments and make predictions, allowing for a clear understanding of how different factors interrelate. However, they share similar pitfalls with classification trees, particularly concerning overfitting. They may present overly complex models that do not generalize well to unseen data.
It's essential to regard both classification and regression trees as complementary tools in the decision-making toolkit for businesses. Understanding their structure, components, and appropriate applications enhances their effectiveness, leading to informed and strategic decisions.
Decision Trees in Business Settings
Decision trees offer a structured and visually intuitive way to approach complex decision-making processes. In the fast-paced world of business, where choices can have significant financial implications, employing decision trees can simplify the chaos. They help break down intricate problems into smaller, manageable parts, promoting clarity and aiding in strategic analysis.
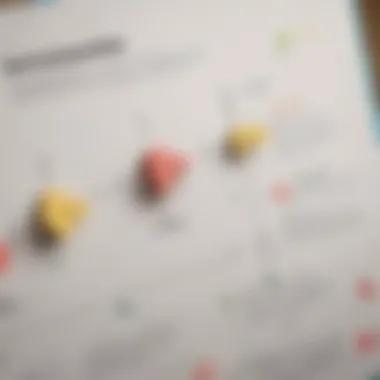
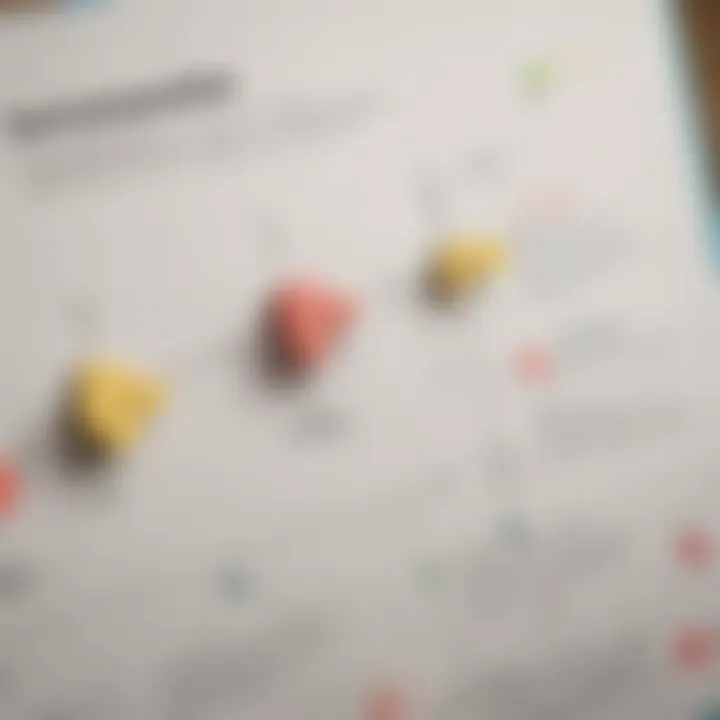
The importance of decision trees in business settings cannot be overstated. These tools assist in identifying various possible outcomes associated with different actions. By laying out options clearly, teams can aim at grounded decision-making processes that support better overall business outcomes. Some key benefits include:
- Visualization: Decision makers can visualize the impact of their choices, making it easier to understand potential outcomes.
- Risk Assessment: They allow for a detailed examination of risks associated with different decisions, a critical aspect in sectors like finance and healthcare.
- Facilitated Communication: When discussing strategies, decision trees provide a common language and comprehensive view for stakeholders.
Perfectly tailored for dynamic industries, decision trees highlight the importance of effectively analyzing data before making decisions. The various use cases in sectors like healthcare, finance, and retail can further illustrate their versatility.
Use Cases in Various Industries
Healthcare
The healthcare sector stands out as a prime example where decision trees can significantly impact patient management. Specifically, they can play a pivotal role in patient diagnosis protocols. By guiding healthcare professionals through a series of logical stepsâsuch as presenting symptoms, suggesting tests, and recommending treatmentsâdecision trees enhance diagnostic accuracy.
The adaptability of healthcare decision trees is one of their key characteristics. They allow for continuous updates based on emerging research, ensuring practitioners have the latest information at their disposal. This responsiveness can lead to timely interventions, ultimately improving patient outcomes. However, while utilitarian, healthcare decision trees must be tailored carefully to avoid information overload, which could confuse rather than clarify.
Finance
In the finance sector, decision trees help establish investment decision frameworks. Here, they streamline the often convoluted processes of evaluating different investment options. A decision tree can enable investors to visualize returns based on varying conditions, which presents a clearer picture of potential financial gains or losses.
The key characteristic of decision trees in finance is their quantitative nature. Unlike other decision-making aids, they are highly data-driven and provide empirical analytical value, making them a popular choice in the realm of finance. This focus on data can also be a double-edged sword, as complex financial models can lead to overfitting, thus limiting their predictive accuracy in dynamic markets.
Retail
In the retail space, decision trees can serve as a valuable tool for customer segmentation strategies. By analyzing shopping habits and preferences, decision trees allow retailers to classify customers into meaningful groups. This classification can drive targeted marketing campaigns, ultimately boosting sales and customer satisfaction.
One of the distinct features of using decision trees in retail is their ability to adapt to vast amounts of unsorted data. Retailers can thus leverage consumer behavior analytics effectively. However, they must guard against relying too heavily on dated data; stale data can skew results and misguide marketing efforts.
Strategic Planning and Decision Making
Strategic planning benefits immensely from decision trees, which help businesses align their objectives with their initiatives. By integrating decision trees into strategic discussions, teams can prioritize projects based on potential impact and feasibility. This structured approach facilitates better resource allocation and ultimately leads to more informed leadership decisions.
In developing a robust decision framework, organizations often face uncertainties regarding future market conditions or customer preferences. Decision trees help elucidate these uncertainties by providing a clear picture of possible flows and outcomes, allowing decision-makers to weigh their options with confidence.
Example of Decision Tree in Healthcare
In the realm of healthcare, decision trees serve as indispensable tools that can streamline diagnosis and treatment planning. Their utility lies not only in simplifying complex clinical decisions but also in enhancing patient outcomes through standardized protocols. By visually mapping out possible pathways based on symptoms, medical history, and treatment options, decision trees bridge the gap between theoretical knowledge and practical application.
Patient Diagnosis Protocols
When it comes to diagnosing patients, decision trees can be a game changer. Letâs say a patient walks in with a cough. A doctor could use a decision tree to systematically navigate through various questions and possible conditions. The first branch might split into categories like "acute" or "chronic". From there, acute may further divide into causes like "viral infection," "bacterial infection," or "allergic reaction." The tree keeps branching until reaching a likely diagnosis, effectively narrowing down the possibilities.
This method is crucial because:
- It provides a clear visualization of diagnostic choices.
- It reduces cognitive overload, enabling healthcare providers to focus on patient care.
- It ensures consistency in practice, minimizing variations in diagnosis.
By employing decision trees for patient diagnosis, doctors can make well-informed decisions quickly.
Cost-Benefit Analysis of Treatments
Another essential application of decision trees in healthcare is in assessing treatment options. Letâs consider a situation where a patient diagnosed with diabetes has to choose between lifestyle changes and medication. A decision tree can outline the costs and benefits attributable to each option, aiding in patient education and shared decision-making.
For example, one branch may present lifestyle changes, detailing potential benefits like improved health metrics and reduced long-term costs. The other branch can present medication, along with its efficacy and side effects.
This method also allows:
- Clarity of Options: Patients can see how different treatments impact their health and finances.
- Informed Decisions: By illustrating costs versus outcomes, patients are more likely to participate actively in their treatment plans.
- Risk Management: Decision trees can incorporate statistical probabilities, helping patients understand risks associated with each option.
Through thorough analysis and visualization of treatment paths, decision trees enhance the conversation between healthcare professionals and patients, paving the way for better-informed decisions.
In sum, the application of decision trees in healthcare offers a dual benefit: improving the accuracy of diagnoses while also ensuring that treatment decisions are made transparently and collaboratively.
Example of Decision Tree in Finance
In the financial realm, decision trees serve as a valuable asset for various analytical undertakings. They allow professionals to systematically evaluate potential outcomes based on a range of variables, which is crucial when tackling complex issues that could affect investment portfolios or risk management trajectories. As the financial markets are rife with uncertainty, decision trees facilitate clearer insights by offering a structured decision-making process. This provides a well-laid plan that can adapt to changing circumstances, making finance less of a guessing game and more of an informed science.
Investment Decision Frameworks
When it comes to allocating funds, financial experts often face numerous variablesâmarket trends, interest rates, economic forecasts, and geopolitical factors all play a part in the decision-making process. Utilizing an investment decision tree can illuminate these pathways, creating a roadmap through a sometimes foggy investment landscape.
A potential investment decision tree might include:
- Nodes for critical decision pointsâlike choosing between stocks, bonds, or real estate.
- Branches that depict the possible outcomes of each choice based on historical data and predictions.
- Leaves at the end of branches representing the final decision or outcomeâreturn on investment, potential losses, or market entry strategies.
For instance, if a financial analyst is considering investing in a tech startup, the decision tree can illustrate pathways based on successful or failed product launches, varying levels of consumer interest, or even regulatory hurdles. By laying out each possible scenario, investors can weigh risks and benefits more effectively than by mere instinct or opinion.
"A decision tree provides clarity in a world where financial choices can often lead us down rabbit holes."
Risk Assessment Models
Risk management is an inescapable aspect of finance. Decision trees can be instrumental in this domain, helping analysts evaluate potential risks associated with various financial decisions. They allow for the identification of high-risk versus low-risk propositions and clarify the implications of various choices.
For instance, a financial institution might use a decision tree to evaluate loan applications. Each node could represent factors such as credit scores, income levels, or existing debts. By branching out from these nodes, the decision tree can present outcomes such as:
- Loan approval likelihood
- Expected time for repayment
- Probability of default
With this approach, financial bodies can better manage their risk exposure while making informed choices about approving loans. As the tree grows more complex with additional data points, it leads to a more nuanced understanding of riskâsomething that is invaluable in todayâs intricate financial landscape. Decision trees thus allow for a careful calibration of risks, ensuring that financial strategies are robust yet adaptable to new information.
Example of Decision Tree in Retail
Retail is a dynamic arena where decision-making plays a pivotal role in determining the success or failure of a business. Using decision trees effectively in retail enables stakeholders to visualize options, evaluate risks, and strategize based on data-driven insights. They simplify complex decisions by breaking them down into various potential outcomes, all while highlighting the likely impacts of each choice. This structured approach not only improves clarity but ensures that decisions are backed by solid logic and data.
When considering the retail landscape, two pivotal applications of decision trees emerge prominently: inventory management and customer segmentation. Each serves a distinct yet equally essential purpose in enhancing operational efficiency and boosting sales performance.
Inventory Management Decisions
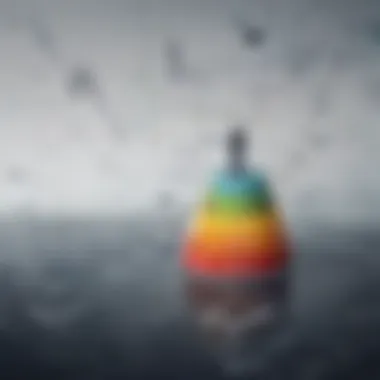
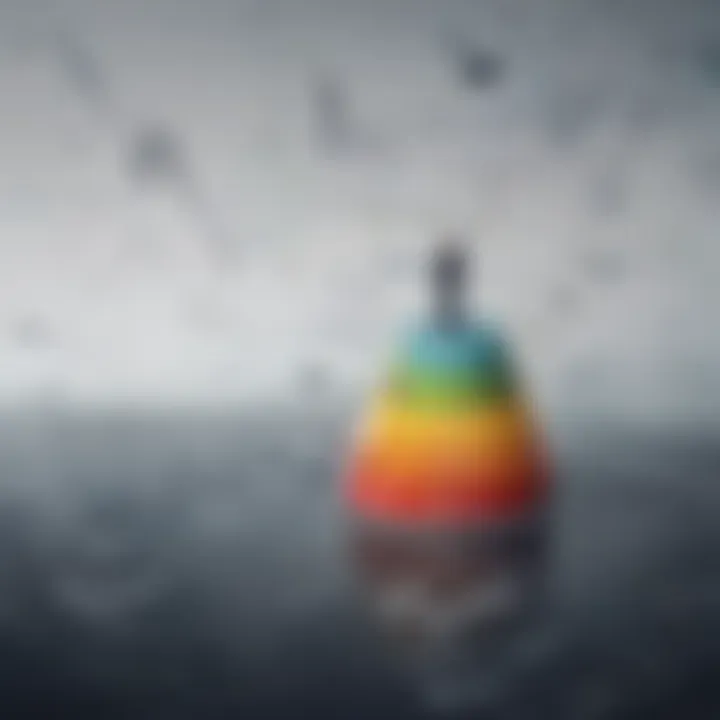
Maintaining the right levels of inventory is a balancing act retail businesses constantly juggle. Too much stock can lead to increased holding costs, while too little can mean missed sales opportunities. By employing a decision tree for inventory management, retailers can navigate these challenges more effectively.
- Demand Forecasting: A decision tree can help in predicting sales based on seasonal trends and historical data, guiding restocking decisions.
- Safety Stock Levels: Retailers can use decision trees to determine optimal safety stock based on factors like lead times and demand variability.
- Supplier Selection: When deciding which supplier to engage, a decision tree can weigh factors like price, reliability, and quality to select the best option.
Moreover, implementing a well-structured decision tree allows businesses to visualize various outcomes associated with their inventory choices. For instance, if the demand exceeds projections, a quick pivot can be made to secure additional stock from alternate suppliers, mitigating potential losses.
Using a decision tree in inventory management makes it easier to see the big picture and ensures retailers are not caught flat-footed in this fast-paced environment.
Customer Segmentation Strategies
Another critical application of decision trees in the retail sector is for customer segmentation. Understanding various customer demographics allows retailers to tailor marketing efforts, optimize stock levels, and enhance overall customer satisfaction.
Hereâs how decision trees can enhance segmentation:
- Defining Customer Profiles: By inputs such as age, purchasing history, and preferences, decision trees help to categorize customers into actionable segments.
- Targeted Marketing Campaigns: After identifying segments, retailers can easily plot out personalized marketing strategies based on the preferences of each group to increase engagement.
- Product Recommendations: Unpacking customer behavior lets brands recommend products that align with each segmentâs past purchasing patterns.
Employing decision trees for customer segmentation paves the way for more precise marketing strategies. This style of targeted approach not only maximizes marketing budget efficiency but also fosters a stronger customer relationship by delivering personalized experiences.
In summary, decision trees in retail not only streamline decision-making but also fortify strategic initiatives aimed at enhancing operational efficiency and customer satisfaction. By embracing this analytical tool, retail stakeholders are better equipped to navigate the complexities of their industry and achieve robust outcomes.
Benefits of Using Decision Trees
Decision trees stand out as a significant tool in business, offering noteworthy advantages that can transform decision-making processes. They simplify the complexities inherent in data analysis and strategic planning. By turning nuanced datasets into a visual format, decision trees enable a more straightforward interpretation of information.
Here are the primary benefits of using decision trees in a business context:
- Visual Representation: The graphical nature of decision trees helps stakeholders grasp the implications of decisions quickly. This visual layout not only aids in understanding individual choices but also their potential outcomes.
- Intuitive Decision-Making: With decision trees, the path to a conclusion feels more like a journey rather than a labyrinth. It presents a clear route that reflects the decision criteria, resulting in less confusion.
- Structured Data Analysis: Decision trees impose a structured approach to analyzing data, allowing organizations to recognize patterns, trends, or inconsistencies that may otherwise fly under the radar.
- Cost-Effectiveness: The insights generated from decision trees can lead to more cost-effective decisions. For instance, in healthcare, a precise treatment pathway can avoid unnecessary expenses.
The relevance of this topic cannot be overstated. As businesses navigate ever-changing landscapes, these decision-making aids become crucial in realigning strategies and managing risks, ensuring they remain perched on the edge of innovation.
Enhanced Clarity in Decision Making
When facing crucial decisions, clarity tends to be a well-worn path to success. Decision trees provide that clarity by breaking down choices into comprehensible segments. Imagine a scenario where a company must determine whether to launch a new product line. A decision tree allows the team to evaluate various factors, such as market demand, costs, and potential competition.
Each branch of the tree represents different alternatives, leading to possible outcomes. This clear setup means team members can readily compare and contrast options, leading to more informed decisions. Key points include:
- Easier Assessment of Risks: Businesses often wrestle with uncertainty. Decision trees help illustrate and evaluate potential risks associated with various choices, allowing teams to mitigate them proactively.
- Consistent Decision Processes: By providing a standardized approach for making decisions, they ensure uniformity in analyses. This can establish a strong foundation for ongoing strategy implementation across teams and departments.
Facilitated Communication of Complex Scenarios
In the world of business, communication is vital yet challenging, especially when discussing intricate scenarios. Here, decision trees shine brightly. They provide a shared language through visuals that everyone can understand, regardless of their level of expertise.
When you use a decision tree to depict a complex problemâsay, risk assessment in an investment strategyâeach partition defines different risk scenarios. This clearly illustrates how different decisions could lead to various risk levels, fostering open discussions.
Consider these advantages of facilitated communication through decision trees:
- Transparency in Decision Processes: Stakeholders can see how decisions are made, creating an environment of trust. When each stage and its associated consequences are laid out visibly, accountability increases.
- Guided Discussions: Instead of delving into abstract language or convoluted statistics, decision trees facilitate focused conversations centered on actual paths and outcomes.
- Engagement Across Teams: By encouraging participation from diverse departments, the decision-making process can benefit from a wider range of perspectives. This collaborative effort often leads to wiser decisions.
Ultimately, leveraging decision trees can demystify complex decision-making processes. They pull everyone onto the same page, eliminating confusion and enhancing mutual understanding among team members and stakeholders.
Limitations of Decision Trees
Every tool has its edges, and decision trees are no exception. While they offer substantial benefits in terms of clarity and structuring complex decisions, certain limitations can hinder their effectiveness in various business applications. It's crucial to understand these drawbacks because they shape how decision trees should be implemented and interpreted. Recognizing these limitations not only helps in avoiding common pitfalls but also guides business professionals in selecting the right decision-making tools for their scenarios.
Overfitting Issues
One of the primary concerns related to decision trees is overfitting. This occurs when a tree model learns not just the underlying patterns in the training dataset but also the noise. Consequently, while the tree may perform remarkably well on the training data, its performance can plummet on new, unseen data, leading to poor predictions.
For instance, think about a restaurant deciding whether to add a specific dish based on last monthâs sales data. If the decision tree accounts for every little fluctuation in the daily sales (like a particularly busy Tuesday), it might recommend that dish solely based on that temporary spike. In reality, that spike could have been an anomaly rather than a trend.
To mitigate overfitting, practitioners often resort to techniques like pruning, which involves removing sections of the tree that provide limited power in predicting outcomes. Another approach is to set constraints on the tree's depth, ensuring it remains general enough to apply to new data without locking in on specific variances from the training set.
Challenges in Handling Uncertainty
Decision trees usually operate under the assumption that data is well-structured and that the relationships among variables are clearly defined. However, in real-world situations, uncertainty frequently rears its head. For instance, market fluctuations, changes in consumer behavior, or unexpected global events can introduce variables that are not accounted for in the initial model.
Consider a finance company evaluating potential investments through a decision tree. If the investment decision hinges heavily on factors that can change overnightâlike regulations or economic indicatorsâthe model may provide misleading guidance. This reliance can lead to costly decisions based on what should have been a more exploratory approach, rather than a definitive pathway.
To combat these issues, businesses must incorporate uncertainty into their decision trees. Techniques such as sensitivity analysis can help gauge how variations in one or more input variables can affect the predictions. Additionally, integrating probabilistic models with decision trees enables a more flexible approach, allowing organizations to prepare for various scenarios rather than leaning too heavily on a single forecast.
"Every choice reflects the balance between certainty and uncertainty, and decision trees must adapt to this dance."
Best Practices for Decision Tree Implementation
When it comes to deploying decision trees in business applications, following best practices can mean the difference between a model that thrives and one that falters. Implementing these practices allows organizations to harness the full potential of decision trees, leading to sounder decisions and better results.
Data Collection and Preparation
Before you dive into using decision trees, the groundwork must be laid through comprehensive data collection and preparation. Without high-quality data, even a sophisticated decision tree can produce skewed results.
First, understand your data sources. Are they reliable? Accurate? This is key. If your inputs are questionable, the output will mirror those flaws. Businesses often overlook this step, assuming any data is good enough. In fact, it might backfire.
Next, perspective on data preprocessing is crucial. This means cleaning your dataâremoving duplicates, addressing missing values, and standardizing formats. You might consider normalization or standardization to ensure that the scales of different variables do not distort the decision-making process.
In addition, feature selection comes into play. It is not uncommon to have a bucketload of features, but not all of them will hold up under scrutiny. You want to zero in on the most relevant variables that drive the decision treeâs effectiveness. Techniques like Feature Importance or Recursive Feature Elimination could be handy tools in this stage.
Finally, segment your data into training and testing sets. This helps in validating the performance of your decision tree. In the end, the effort you put into this preparatory phase will greatly enhance the modelâs efficacy.
Choosing the Right Type of Tree
The choice of tree type is pivotal in dictating how well your desired outcomes align with the modelâs performance. The two main typesâclassification trees and regression treesâare designed for different scenarios.
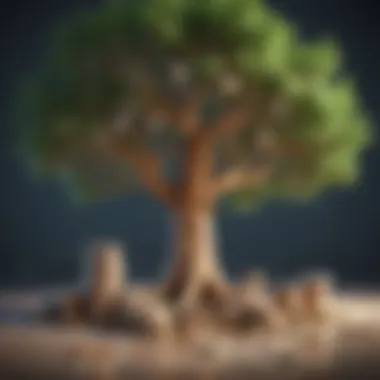
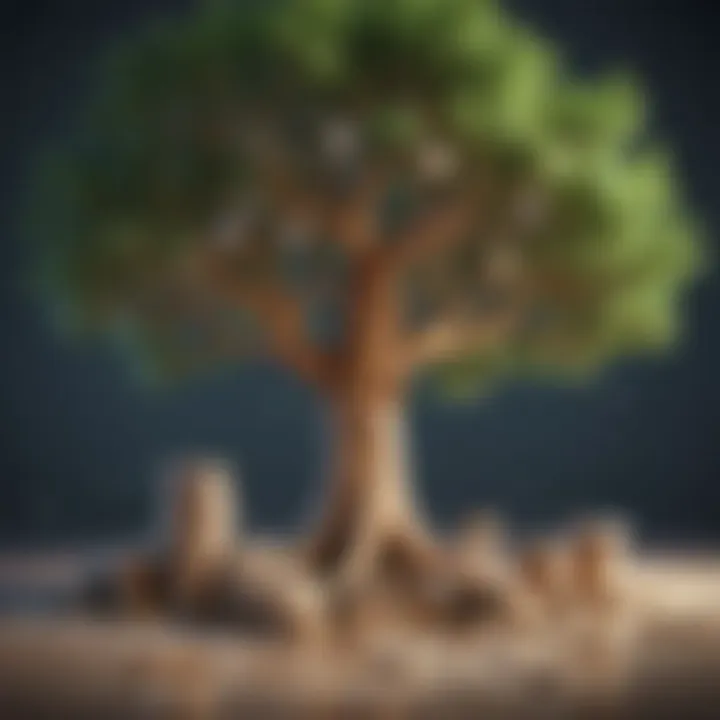
Classification trees are ideal when the outcome youâre predicting is categorical. For example, determining whether a loan applicant is creditworthy or risky falls under this umbrella. These trees aim to classify or categorize outcomes based on predefined labels.
On the other hand, regression trees are the way to go when dealing with continuous outcomesâsuch as estimating the future sales of a product. In this case, the tree helps predict numerical values rather than categories.
Once youâve identified the type of decision tree relevant to your objective, another key consideration is determining decision tree depth. A deeper tree can capture more complex relationships but runs the risk of overfitting. Maintaining a balance here is crucial.
Your target audience, whether investors, financial advisors, analysts, or students, should carefully assess the decision tree type against the specific business application for optimal results.
"Understanding what kind of decision tree a business needs is like picking the right tool for the job; using a screwdriver where a hammer is required wonât yield any results."
In wrapping up this section on best practices, remember that knowledge is power when it comes to implementing decision trees. Preparation and the right choice of model will serve as your compass in navigating the complexities of business decisions.
Integrating Decision Trees with Other Methods
Decision trees are a valuable tool on their own, but their power can be significantly enhanced when combined with other methods. This integration may improve accuracy, enrich data analysis, and lead to more meaningful insights in decision-making processes. Utilizing decision trees alongside statistical techniques and machine learning approaches allows businesses to tap into a broader range of data and modeling capabilities. Understanding how these integrations work is vital for investors, financial advisors, analysts, and students to navigate todayâs complex business landscape effectively.
Combining with Statistical Techniques
Integrating decision trees with statistical techniques allows for deeper insights during the data analysis phase. Techniques such as regression analysis can provide a solid foundation for decision trees, especially when dealing with continuous outcome variables. For instance, one might opt for a linear regression to predict sales and then apply a decision tree to categorize the data based on various customer behaviors.
This combination can highlight correlations that might have gone unnoticed if decision trees were used in isolation. By using statistical analysis first, businesses can:
- Identify significant predictors much before constructing the decision tree.
- Quantify relationships and influences among different variables cited in the decision path.
- Validate the assumptions underlying the outputs of decision trees.
Moreover, statistical techniques can facilitate robust feature selection for the tree, ensuring that only the most impactful variables influence the decision-making process.
"Integrating traditional statistical methods with decision trees leads to a more nuanced understanding of the data landscape, helping companies better align their strategies with real-world dynamics."
Using Machine Learning Approaches
The marriage of decision trees and machine learning techniques has transformed the way predictions and classifications are made. In essence, decision trees can serve as a building block for more complex algorithms such as Random Forests or Gradient Boosting Machines. These advanced methods often leverage multiple decision trees, enhancing prediction accuracy through ensemble learning.
When machine learning is integrated with decision trees, companies can realize multiple benefits:
- Handling Large Datasets: Machine learning algorithms excel when it comes to processing large volumes of data, making them an excellent fit for businesses sitting on heaps of unstructured or semi-structured data.
- Increased Predictive Power: By using logistic regression or support vector machines in tandem with decision trees, firms can make their forecasting efforts more reliable.
- Improved Generalization: Decision trees are prone to overfitting, but combining them with machine learning techniques can mitigate this issue, leading to models that generalize better to unseen data.
This integration may not be without challenges; thus, businesses need to keep considerations such as computational efficiency, model interpretability, and data quality in mind. Striking a balance between complexity and usability is crucial as firms aim for actionable insights without getting mired in misunderstandings.
Real-World Case Studies
The exploration of real-world case studies provides invaluable insights into how decision trees operate within business applications. These case studies serve as practical exemplars for organizations aiming to enhance their decision-making processes through structured approaches. They underscore the adaptability of decision trees across industries and showcase the tangible benefits of incorporating such methodologies into operational frameworks. By dissecting specific real-world implementations, the reader can grasp the nuances of decision trees and their significance in driving successful outcomes.
Case Study in Healthcare Management
In the realm of healthcare, decision trees play a pivotal role in patient management and treatment planning. Consider, for instance, a healthcare facility that utilizes a decision tree to streamline its patient diagnosis protocols. A hypothetical case could involve a hospital employing a decision tree to determine whether a patient presenting with certain symptoms should receive further testing for illnesses like diabetes or thyroid dysfunction.
The decision tree starts at the initial symptoms, branching into various paths based on patient age, family history, and other relevant factors. Each branch draws further into more specific symptoms or lab results. This approach enables healthcare professionals to make informed decisions swiftly, ensuring that critical resources are allocated effectively.
The outcome not only enhances patient care but also optimizes resource utilization. By assessing which tests are necessary based on a patientâs history and symptoms, hospitals can reduce unnecessary tests, saving costs and time for both patients and providers. A decision tree can also continuously evolve, learning from new cases to refine and improve diagnostic accuracy over time. This aspect of adaptability is crucial in a field where patient data is always changing.
Case Study in Financial Services Optimization
Turning to the finance sector, decision trees provide essential frameworks for optimizing various processes, including credit risk assessment. A notable example could involve a lending company applying decision trees to evaluate loan applications. A real-world scenario illustrates how a financial institution might approach approving loans using a model that incorporates decision trees.
The process begins with key inputs like credit score, income level, and past financial behaviors. From there, a decision tree branches out, leading to various outcomes depending on risk factors. If a consumer has a high credit score but unstable income, the tree can flag a higher risk, suggesting either a higher interest rate or a need for additional documentation.
Such structured assessments help lenders make equitable and swift decisions while maintaining financial prudence. Moreover, these tools can be integrated with machine learning algorithms, allowing them to refine their predictive capabilities. As a result, not only does the financial institution minimize the risks associated with lending, but it also enhances their customer service experience by accelerating the processing time of applications.
Research indicates that decision trees can improve accuracy by 10-15% compared to traditional assessment methods, showcasing their effectiveness in real-world applications.
Future Trends in Decision Tree Applications
In the ever-evolving landscape of business, the integration of decision trees is undergoing significant transformation, largely driven by technological advancements. As organizations grow and face increased complexity in their operations, the relevance of predictive analytics becomes pronounced. Decision trees, with their intuitive structure, stand at the forefront of this development, offering clarity amid chaos.
Advancements in AI and Machine Learning
The marriage of decision trees with artificial intelligence and machine learning is reshaping how businesses approach data-driven decision making. This combination enhances predictive accuracy and provides deeper insights that were previously unattainable. Machine learning algorithms refine decision tree models through techniques such as boosting and bagging, allowing for more robust outputs.
For instance, health insurance companies can now use advanced decision trees that incorporate patient data with AI to determine the potential health risks associated with specific demographics, resulting in more tailored insurance products. This proactive approach not only strengthens client relationships, but also optimizes underwriting processes.
In financial sectors, AI-enhanced decision trees analyze customer transaction history and identify patterns that may indicate fraud. This not only speeds up the detection process but also reduces the number of false positives, saving institutions considerable resources.
Evolving Business Needs and Decision Support Systems
As businesses pivot to meeting dynamic market demands, the design of decision support systems (DSS) must evolve correspondingly. Here, decision trees demonstrate versatility, adapting to the changing frameworks of business needs. Companies today seek solutions that can react quickly and effectively to data flows that arrive in real time.
- Agricultural tech has carved new ground by employing decision trees in crop management systems, guiding farmers based on weather patterns, soil health, and market price fluctuations.
- Retail giants, using sophisticated decision trees, segment customers not just by demographics but also by purchasing behaviorâproviding tailored recommendations and enhancing user experience.
"Decision trees are not just a tool; they are a compass navigating the complexities of modern business landscapes."
Integrating these decision trees into broader decision support systems ensures that organizations are not merely reacting to data but are strategically leveraging information for foresight. As such, staying ahead in the game will require businesses to invest in technologies that blend human intuition with data science, ensuring that their decision-making frameworks are as efficient and informed as possible. This tailored approach to decision-making empowers companies to not only respond to but anticipate changes in their respective sectors, ultimately driving better results.
The End
In the world of business, making sound decisions can often feel like navigating a labyrinth. This article has explored how decision trees serve as invaluable tools, illuminating the path forward amidst complexities. With their structured yet flexible nature, decision trees aid not just in decision-making but also in clarifying processes that can otherwise become a tangled web of uncertainties.
Understanding the key takeaways from the use of decision trees can enhance their practical application significantly. Decision trees help streamline strategic planning, support risk assessment, and facilitate effective communication among stakeholders. In particular circumstances, they lay out the possibilities in an easy-to-follow format, allowing both seasoned professionals and newcomers to grasp intricate details quickly.
Summarizing Key Takeaways
- Clarity in Complex Scenarios: Decision trees break down complicated scenarios into manageable segments, offering clear insight into various choices and their consequences.
- Industry Versatility: They find utility across a range of sectors, such as healthcare and finance, catering to distinct needs while remaining adaptable.
- Risk Management Tools: By assessing potential outcomes, decision trees empower organizations to mitigate risks more effectively during decision-making processes.
- Collaboration & Communication: The visual nature of decision trees enhances communication, making it easier for teams to collaborate on strategic efforts and shared goals.
Final Thoughts on Decision Trees in Business
Integrating decision trees into business frameworks is not merely about adopting a tool but is rather about embracing a mindset that supports informed decision-making. As trends shift, particularly with advancements in data science and machine learning, the role of decision trees is evolving but remains foundational in guiding effective strategy.
As evidenced throughout this article, decision trees are far more than just diagrammatic representations of choices; they stand as a robust framework for navigating the often murky waters of business decision-making. By fostering transparency, driving clarity, and supporting strategic goals, decision trees can position organizations to thrive in an ever-changing landscape.
Ultimately, as businesses continue adapting to new challenges and opportunities, understanding how to leverage decision trees is essential. As these techniques continue to advance, staying abreast of developments promises not only to enhance decision-making processes but to uncover new avenues for growth, efficiency, and success.